There’s a reasonably established school of thought that suggests the world, it’s machines, its cities and its products have all become too complex for the human brain to comprehend. Managing transport systems, for example, like roadways or airports can’t be done efficiently anymore by human beings… at least not alone.
If you’re an engineer and you don’t understand that the rules and tools of the game are changing, you’re going to be left behind in a wake of disruptive technologies. Though the term is frequently overused by just about everyone, society is on the brink of being driven by a new fuel. Oil is being replaced by data. And the most valuable firms on the planet – Amazon, Facebook, Google and Microsoft – are furiously mining the new commodity, using the cloud as their pipeline.
The consumer-based Internet of Things and Industry 4.0 are also all about data acquisition, and the sheer amount of data that’s due to be captured is staggering. But data, itself, has little value. What has changed is that there is a fast emerging enabling technology that can find hidden insight and value within it. That technology is artificial intelligence (AI) and the application of AI known broadly as machine learning. AI is set to fundamentally change the world as we know it, but also how engineers think about and tackle problems.
“Our tools and what we expect from them are undergoing a metamorphosis,” says Mike Haley, the senior director of machine learning at CAD giant Autodesk. “They are being transformed by abundant computation. When computation was finite, we made tools that need input and direction. You directed the tools. But now, we can flip the whole thing around. No longer simulating based on a design. And not just simulating during design. We can simulate to discover design.
“Generative design tools anticipate our needs, and in doing so, help us discover the best solution possible. For generative design to work, you describe the problem. What are the goals, the constraints and the forces at play? These new tools help you discover what is possible by using abundant compute power to analyse the entire solution space, discovering ideas you would never have come up with.”
To understand why AI can find ‘a better solution’ we have to first understand our own very human limitations. Most products, if you look hard enough, are embedded with human fallibility, which has been slowly absorbed through years of inherited design and poor iteration. It means that what we think is an optimised solution is often riddled with needless compromise.
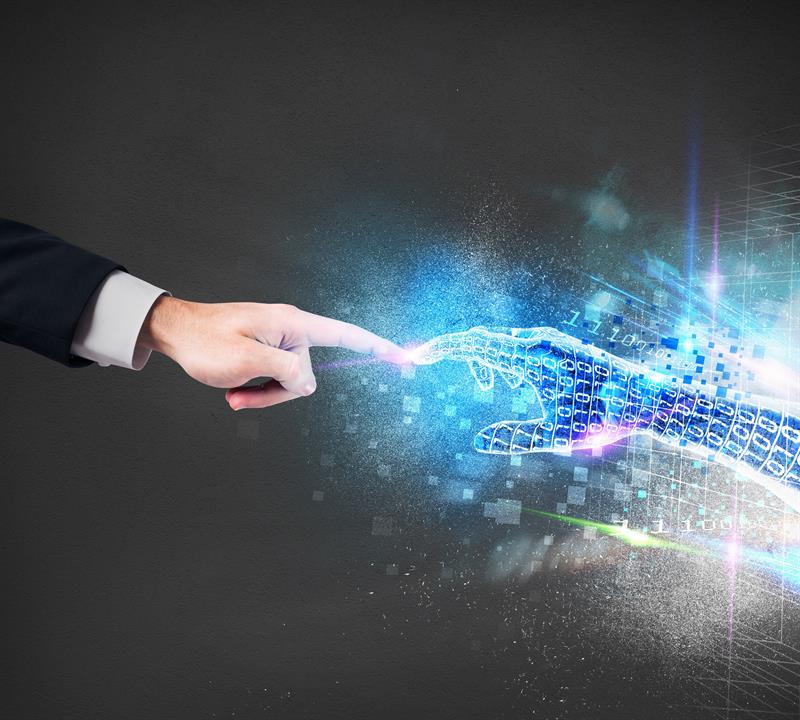
Design psychology and bias
There are about 250 documented human biases and we all have them. Even when we are aware of them, we can’t help but use them as they are intrinsic to our psyche. From an evolutionary perspective, bias allows us to deal with complexity. Bias, or ‘gut-feel’, points to a solution.
“When we come up against an everyday problem, we don’t sit there, shocked, thinking ‘there are a million possible solutions, which one will I choose?’” continues Haley. “You immediately think of just a few as the brain automatically funnels down the options. But those might not be optimal.
“Bringing AI techniques into design tools means you don’t need to worry about your bias. You can let the tool guide you.”
The idea is that instead of defining geometry, engineers will define boundary conditions. It is about telling the software the function that is required, and then letting the software come up with an optimised solution. Broadly known as generative design, the software assesses thousands of design possibilities that an engineer, or even team of engineers, would never be able to achieve. And while a certain design concept might have been dismissed at an early stage, AI might find that actually if you did proceed down that road and overcome ‘x’ then it is a far better solution overall. Think 48v car batteries, for example. AI is the ability to start each project with a clean sheet of paper and from first principles. It is not about experience or prejudice, but assessing physics and performance.
“When it comes to configuring systems architecture, machine learning itself can already do it better than we can,” says Haley. “Take Google Translate, which we use to translate directly from one language to another, like a tourist using a phrase book. That explains why some of the results can be a little off.
“But when the architecture of Google Translate was subjected to a machine learning system, it yields a totally different result. One which today can absorb the meaning from any language into a central language neutral representation of ideas, which in turn can be expressed in any other language. This preserves so much more of the nuance of language. The downside is that no one knows how it works. It’s inscrutable. The machine design complexity escapes our comprehension.”
Machine learning
Machine learning algorithms allow computers to do just that, acquire insight based on the data that they are receiving. It goes well beyond the standard IF/THEN commands used in classical programming. Instead programs are able to become predictive and focus on principles and not mathematical formulas.
The technique is being applied in a number of areas including facial recognition, cancer detection and driving, with progress making solid headway. But, this is the tip of the iceberg.
Stan Schneider, the CEO of Real-Time Innovations, a company that claims to live at the intersection of functional artificial intelligence and pervasive networking, says: “Has anyone done the Math of Moore’s law? How much better do you think a computer will be in 40 years? Computers, today, can almost drive and write music as well as we can. But, computers 40 years from now, if you do the Moore’s law calculation, aren’t going to be 100 times better, a 1000 times better or a million times better… they will be a mind boggling 100 million times better than they are today.
“So, your kids are not going to drive, they won’t compose music and they won’t write… they might for fun but they won’t have to… Machine learning will make us all healthier, greener, smarter and safer.”
Changing tools
Just as machine learning is able to take data and form insights, the tools being developed by some industry players are looking to leverage the expected incoming flow of data. With digital twin capturing terabytes of real world data on a daily basis, a machine learning algorithm will very quickly form ideas and
conclusions about how to design for optimal performance or minimal cost. All it needs are boundary conditions from engineers to specify the requirements.
While this all might sound a bit far-fetched and way off in the future, the technique is already being applied, today.
“We see symbols and algebra signs as ways of manipulating our CAD tools, the computer doesn’t,” says Haley. “Here, data drives the design. And as we capture increasing amounts of data, we can tackle ever bigger problems because of the capability of modern machine learning techniques and massive parallelism of the computing systems that they run on.”
It was recently put to the test by a US research project to investigate ways to apply the technologies to building a performance car. Hackrod wired a car with sensors and then put it through a punishing test drive in the Mojave Desert, California. The result was a massive data set that described the car’s structure, the external environment and all the forces acting on it.
The team then used the data as the ‘boundary conditions’, essentially the environment that the car needed to operate within. This data was plugged into the generative design system, which created hundreds of new options for the frame. The aim was to make it as light as possible, but with the same strength and stiffness.
The result was a frame that was 25% lighter but just as strong as the original. While the frame looks similar to a topologically optimised part, the system was not removing material from the frame, but designing the frame from scratch.
“A human would find that almost impossible to design,” says Chris Bradshaw, a senior vice president at Autodesk. “The next wave of innovation is going to be driven by data. As sensors spread across almost every industry and the Internet of Things, it is going to trigger a massive influx of data that can be used to drive future designs.
“We’ve struggled for decades to analyse and utilise all this data, and data is fairly useless on its own. But, the implications of AI in the design space are enormous.”
Overcoming assumptions
Simulation, historically, has been based around algorithms that essentially calculate real world performance based on numerous assumptions. Nearly all of our models in the world are basic approximations routed in mathematics. And though accurate, the insight that can be gained is entirely at the mercy of the engineer’s skill and their biases. But by teaching an AI with real world data, simulation becomes enabled by intuition and reason rather than long winded calculation and bottom up formulaic results.
“Just as we need to let go of the wheel to realise the potential for self-driving cars, we also need to let go of our traditional tools,” concludes Haley. “Only then will we realise its full potential. Our design tools can remain permanently connected, permanently learning and growing. And so, they transform into something much bigger. What we are seeing is something that is greater than the sum of its parts.
“We need to let go of the tool and let the tool come to us. So, the tools are not only as logical and analytical as ever, but they are also creative and intuitive. At Autodesk, we are designing these tools and we view them with an equality of a true design partner that is not just good at execution, but exploration too.”